What Defines Closed Source Large Language Models? - Understanding Common Characteristics
- Scarlet AI
- Jul 29, 2024
- 3 min read
Large language models (LLMs) like GPT-4, Claude 3.5, and others have revolutionized natural language processing and AI-driven applications. These models, especially those developed by major tech companies, often come as closed source, meaning their underlying code and training methodologies are not publicly available. In this post, we'll explore the common characteristics of closed source large language models and why these features are integral to their design and functionality.
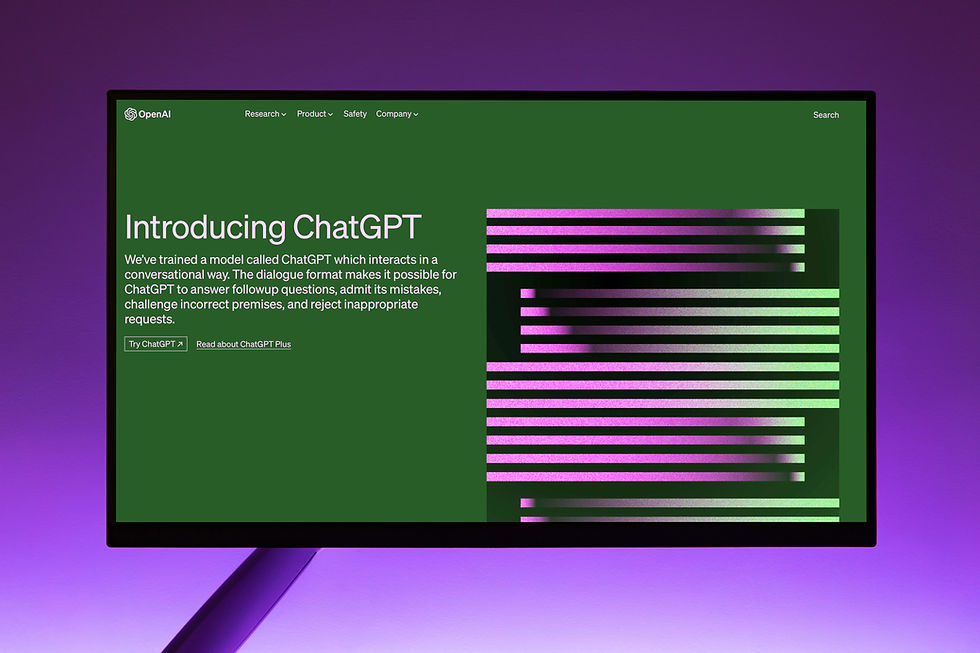
1. Proprietary Technology and Techniques
One of the defining characteristics of closed source large language models is the use of proprietary technology and techniques. These models are developed using unique algorithms, training methods, and data preprocessing techniques that are not disclosed to the public. This proprietary approach allows companies to maintain a competitive edge by leveraging advanced technologies that are not easily replicable.
Example: OpenAI's GPT series and Anthropic's Claude models are based on proprietary architectures and training protocols that distinguish them from other models. The exact details of their neural network designs, data filtering processes, and optimization strategies are kept confidential.
2. Extensive Training Data and Resources
Closed source LLMs are typically trained on vast and diverse datasets that are often unavailable to the public. These datasets include text from books, articles, websites, and other digital content, allowing the models to learn a wide range of language patterns and contexts. The training process requires significant computational resources, often involving thousands of GPUs or TPUs over extended periods.
Example: GPT-4 is trained on a diverse dataset covering numerous languages and topics, enabling it to understand and generate human-like text across a wide range of domains. The scale of training data and computational power used is a key differentiator from open-source models.
3. Emphasis on Commercial Applications
Closed source models are often designed with commercial applications in mind. Companies developing these models aim to integrate them into various products and services, such as chatbots, content generation tools, virtual assistants, and more. This commercial focus drives the development of features that enhance usability, scalability, and integration with existing technologies.
Example: AI models like OpenAI's GPT-4 are integrated into commercial products like ChatGPT, which provides conversational AI services for customer support, content creation, and more. The closed source nature ensures control over the quality and consistency of the deployed models.
4. Focus on Ethical and Safety Considerations
Given the potential impact of LLMs, closed source models often prioritize ethical considerations and safety measures. Companies implement various safeguards to prevent misuse, bias, and harmful content generation. These measures may include filtering training data, fine-tuning models with specific guidelines, and implementing real-time monitoring and moderation systems.
Example: OpenAI has implemented content moderation tools and safety protocols to prevent the generation of harmful or inappropriate content. This involves both pre- and post-deployment safety checks, ensuring the model adheres to ethical standards.
5. Restricted Access and Licensing
Access to closed source LLMs is typically restricted through licensing agreements or API access, rather than providing open access to the model's code or weights. This approach allows companies to maintain control over how the models are used, ensuring compliance with terms of service and preventing unauthorized or harmful applications.
Example: OpenAI offers access to its GPT models via API, with usage governed by specific terms and conditions. This ensures that the models are used responsibly and in line with the company's ethical guidelines.
6. Continuous Improvement and Updates
Closed source models are continuously updated and improved by the developers, often based on user feedback, advancements in AI research, and emerging ethical considerations. This ongoing development process ensures that the models remain state-of-the-art and can adapt to new challenges and requirements.
Example: OpenAI's iterative updates to its GPT models reflect ongoing improvements in performance, safety, and ethical handling of content. These updates are informed by research findings and real-world use cases, ensuring the models evolve in a responsible and effective manner.
Closed source large language models, characterized by proprietary technology, extensive training resources, commercial focus, and stringent ethical standards, play a significant role in the AI landscape. While the closed nature of these models limits public scrutiny and customization, it also enables companies to maintain control over their technology, ensuring quality, safety, and compliance with ethical guidelines.
As these models continue to evolve, the balance between innovation and ethical responsibility will remain a key focus. Understanding the common characteristics of closed source LLMs helps in appreciating the complexities and considerations involved in developing and deploying these powerful tools.
At Innovelle, we specialize in harnessing the power of AI to transform businesses. Whether you're looking to integrate advanced AI language models into your digital marketing strategies or enhance your customer interactions with cutting-edge chatbots, our expert team can help you navigate the complexities of AI technology. Contact us today to learn how Innovelle's AI-driven solutions can elevate your business to new heights!
Comments